Recent Insights
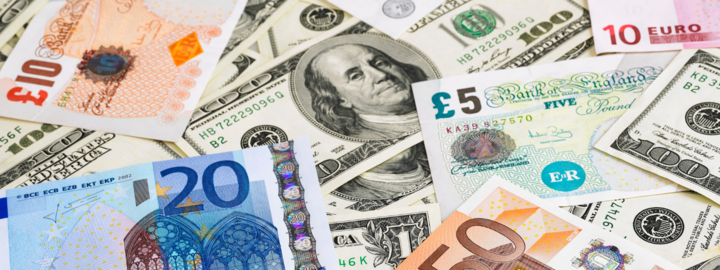
Minority report: Minority stakes spike in US and Europe for sponsors and strategics alike
M&A Explorer | In an M&A market characterized by buyer caution and narrowing liquidity pathways for sellers, flexible minority stakes transactions are proving a valuable option for dealmakers in the US and Europe
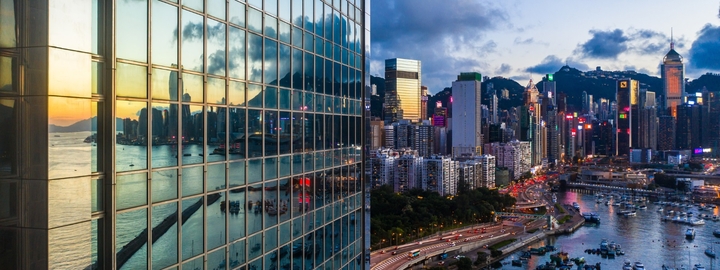
Rising tides: Asia-Pacific PE market grows in the face of global uncertainty
M&A Explorer | APAC private equity markets have entered a holding pattern as firms face global trade and tariff uncertainty, but sophisticated managers are uncovering opportunities for investing in high-quality assets at attractive valuations
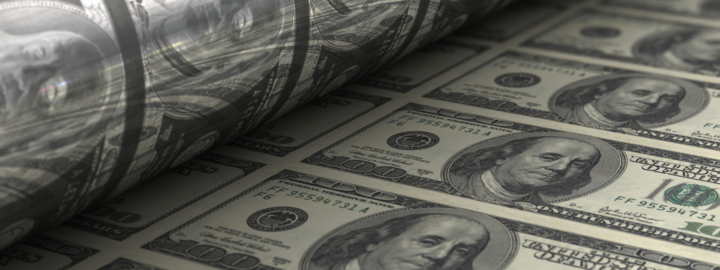
Delayed-draw facilities: A key differentiator in a competitive market
Debt Explorer | Delayed-draw facilities have been a key feature of private credit lending terms, but as competition between private credit and broadly syndicated loans intensifies, broadly syndicated loans have started to incorporate delayed-draw flexibility
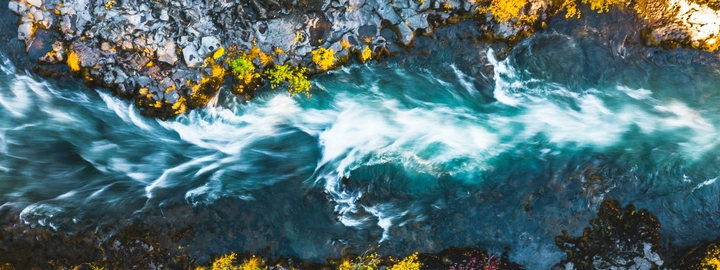
Currents of Capital 2025 Report
Investment trends and opportunities in the global water sector drawing on the perspectives of over 300 senior decision-makers across the global water value chain, including water utilities, multinational corporations, investment funds, engineering firms and technology providers.
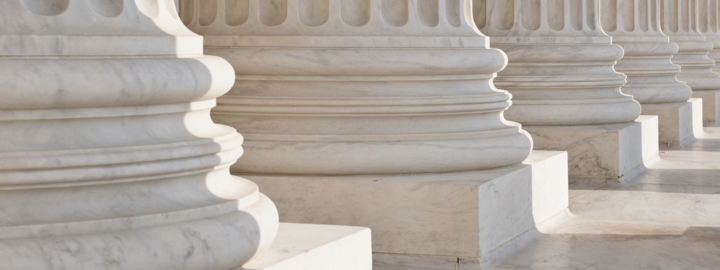
Transatlantic transactions: Uncertainty is the “new normal” for US-Europe M&A, but deals will get done
M&A Explorer | The pro-business agenda of the new US administration looked set to boost outbound M&A into Europe in 2025, but tariff announcements and geopolitical tumult mean that dealmakers are holding fire for now